lm and glm function in R Unicorn Meta Zoo #1: Why another podcast? Announcing the arrival of Valued Associate #679: Cesar ManaraIntuition behind logistic regressionWinning percentage — logistic regression or linear regression?Why do we use logistic regression instead of linear regression?significance of coefficients and significance of marginal effectsIs it possible to model the conditional expectation of a binary outcome using an additively-separable link function?Logistic glm: marginal predicted probabilities and log-odds coefficients support different hypothesesDoes this analysis make sense and can references to similar work be provided?In a count model, how do I predict the change in y if one of my independent variables changes?marginal effects of a GLMMarginal effect of variables - Logistic regression, Boosted tree, and other tree-based modelsInterpreting Marginal Effect ResultsAbout Partial dependence for Poisson GLMUsing variance-covariance matrix of mixed-effects logistic regression to obtain p-values for custom contrasts
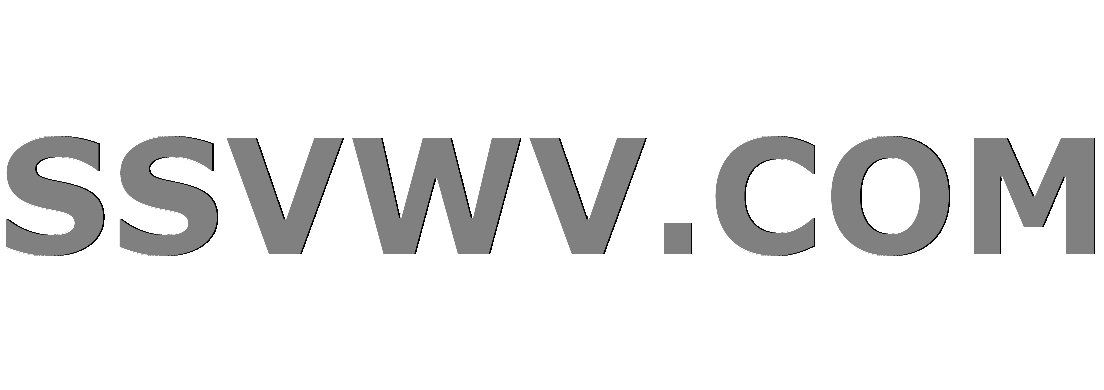
Multi tool use
Israeli soda type drink
How to use @AuraEnabled base class method in Lightning Component?
Are these square matrices always diagonalisable?
"Rubric" as meaning "signature" or "personal mark" -- is this accepted usage?
What's the difference between using dependency injection with a container and using a service locator?
Split coins into combinations of different denominations
Trumpet valves, lengths, and pitch
Is accepting an invalid credit card number a security issue?
Putting Ant-Man on house arrest
How to keep bees out of canned beverages?
Seek and ye shall find
Expansion//Explosion and Siren Stormtamer
"My boss was furious with me and I have been fired" vs. "My boss was furious with me and I was fired"
How to get even lighting when using flash for group photos near wall?
Could moose/elk survive in the Amazon forest?
What’s with the clanks in Endgame?
What to do with someone that cheated their way through university and a PhD program?
My bank got bought out, am I now going to have to start filing tax returns in a different state?
A strange hotel
Co-worker works way more than he should
Suing a Police Officer Instead of the Police Department
Book with legacy programming code on a space ship that the main character hacks to escape
I preordered a game on my Xbox while on the home screen of my friend's account. Which of us owns the game?
Will I lose my paid in full property
lm and glm function in R
Unicorn Meta Zoo #1: Why another podcast?
Announcing the arrival of Valued Associate #679: Cesar ManaraIntuition behind logistic regressionWinning percentage — logistic regression or linear regression?Why do we use logistic regression instead of linear regression?significance of coefficients and significance of marginal effectsIs it possible to model the conditional expectation of a binary outcome using an additively-separable link function?Logistic glm: marginal predicted probabilities and log-odds coefficients support different hypothesesDoes this analysis make sense and can references to similar work be provided?In a count model, how do I predict the change in y if one of my independent variables changes?marginal effects of a GLMMarginal effect of variables - Logistic regression, Boosted tree, and other tree-based modelsInterpreting Marginal Effect ResultsAbout Partial dependence for Poisson GLMUsing variance-covariance matrix of mixed-effects logistic regression to obtain p-values for custom contrasts
.everyoneloves__top-leaderboard:empty,.everyoneloves__mid-leaderboard:empty,.everyoneloves__bot-mid-leaderboard:empty margin-bottom:0;
$begingroup$
I was running a logistic regression in r using glm()
as
glm(Y ~X1 + X2 +X3, data = mydata, family = binomial(link = "logit"))
By accident I ran the model using lm
instead:
lm(Y ~X1 + X2 +X3, data = mydata, family = binomial(link = "logit"))
I noticed that the coefficients from the model using lm()
were a very good approximation to the marginals on the model using glm()
(a difference of $0.005$).
Is this by coincidence or can I use the lm()
as I specify to estimate the marginals for logistic regressions?
marginal-effect
New contributor
Cedroh is a new contributor to this site. Take care in asking for clarification, commenting, and answering.
Check out our Code of Conduct.
$endgroup$
add a comment |
$begingroup$
I was running a logistic regression in r using glm()
as
glm(Y ~X1 + X2 +X3, data = mydata, family = binomial(link = "logit"))
By accident I ran the model using lm
instead:
lm(Y ~X1 + X2 +X3, data = mydata, family = binomial(link = "logit"))
I noticed that the coefficients from the model using lm()
were a very good approximation to the marginals on the model using glm()
(a difference of $0.005$).
Is this by coincidence or can I use the lm()
as I specify to estimate the marginals for logistic regressions?
marginal-effect
New contributor
Cedroh is a new contributor to this site. Take care in asking for clarification, commenting, and answering.
Check out our Code of Conduct.
$endgroup$
$begingroup$
Thank you both for your insight on this issue.
$endgroup$
– Cedroh
2 days ago
$begingroup$
no need to thank us in the comment -- simply up-vote answer you found helpful and select the check mark next to the one that best answered your question. Thanks! As a new user of this site, I just wanted to make sure you knew how to use these functions! You are welcome by the way.
$endgroup$
– StatsStudent
2 days ago
1
$begingroup$
It's a bit of a coincidence that the coefficients were not very different. Among other things, that requires the link function to be nearly the same as the identity function within the range of the explanatory variables.
$endgroup$
– whuber♦
2 days ago
add a comment |
$begingroup$
I was running a logistic regression in r using glm()
as
glm(Y ~X1 + X2 +X3, data = mydata, family = binomial(link = "logit"))
By accident I ran the model using lm
instead:
lm(Y ~X1 + X2 +X3, data = mydata, family = binomial(link = "logit"))
I noticed that the coefficients from the model using lm()
were a very good approximation to the marginals on the model using glm()
(a difference of $0.005$).
Is this by coincidence or can I use the lm()
as I specify to estimate the marginals for logistic regressions?
marginal-effect
New contributor
Cedroh is a new contributor to this site. Take care in asking for clarification, commenting, and answering.
Check out our Code of Conduct.
$endgroup$
I was running a logistic regression in r using glm()
as
glm(Y ~X1 + X2 +X3, data = mydata, family = binomial(link = "logit"))
By accident I ran the model using lm
instead:
lm(Y ~X1 + X2 +X3, data = mydata, family = binomial(link = "logit"))
I noticed that the coefficients from the model using lm()
were a very good approximation to the marginals on the model using glm()
(a difference of $0.005$).
Is this by coincidence or can I use the lm()
as I specify to estimate the marginals for logistic regressions?
marginal-effect
marginal-effect
New contributor
Cedroh is a new contributor to this site. Take care in asking for clarification, commenting, and answering.
Check out our Code of Conduct.
New contributor
Cedroh is a new contributor to this site. Take care in asking for clarification, commenting, and answering.
Check out our Code of Conduct.
edited 2 days ago
duckmayr
1846
1846
New contributor
Cedroh is a new contributor to this site. Take care in asking for clarification, commenting, and answering.
Check out our Code of Conduct.
asked 2 days ago


CedrohCedroh
311
311
New contributor
Cedroh is a new contributor to this site. Take care in asking for clarification, commenting, and answering.
Check out our Code of Conduct.
New contributor
Cedroh is a new contributor to this site. Take care in asking for clarification, commenting, and answering.
Check out our Code of Conduct.
Cedroh is a new contributor to this site. Take care in asking for clarification, commenting, and answering.
Check out our Code of Conduct.
$begingroup$
Thank you both for your insight on this issue.
$endgroup$
– Cedroh
2 days ago
$begingroup$
no need to thank us in the comment -- simply up-vote answer you found helpful and select the check mark next to the one that best answered your question. Thanks! As a new user of this site, I just wanted to make sure you knew how to use these functions! You are welcome by the way.
$endgroup$
– StatsStudent
2 days ago
1
$begingroup$
It's a bit of a coincidence that the coefficients were not very different. Among other things, that requires the link function to be nearly the same as the identity function within the range of the explanatory variables.
$endgroup$
– whuber♦
2 days ago
add a comment |
$begingroup$
Thank you both for your insight on this issue.
$endgroup$
– Cedroh
2 days ago
$begingroup$
no need to thank us in the comment -- simply up-vote answer you found helpful and select the check mark next to the one that best answered your question. Thanks! As a new user of this site, I just wanted to make sure you knew how to use these functions! You are welcome by the way.
$endgroup$
– StatsStudent
2 days ago
1
$begingroup$
It's a bit of a coincidence that the coefficients were not very different. Among other things, that requires the link function to be nearly the same as the identity function within the range of the explanatory variables.
$endgroup$
– whuber♦
2 days ago
$begingroup$
Thank you both for your insight on this issue.
$endgroup$
– Cedroh
2 days ago
$begingroup$
Thank you both for your insight on this issue.
$endgroup$
– Cedroh
2 days ago
$begingroup$
no need to thank us in the comment -- simply up-vote answer you found helpful and select the check mark next to the one that best answered your question. Thanks! As a new user of this site, I just wanted to make sure you knew how to use these functions! You are welcome by the way.
$endgroup$
– StatsStudent
2 days ago
$begingroup$
no need to thank us in the comment -- simply up-vote answer you found helpful and select the check mark next to the one that best answered your question. Thanks! As a new user of this site, I just wanted to make sure you knew how to use these functions! You are welcome by the way.
$endgroup$
– StatsStudent
2 days ago
1
1
$begingroup$
It's a bit of a coincidence that the coefficients were not very different. Among other things, that requires the link function to be nearly the same as the identity function within the range of the explanatory variables.
$endgroup$
– whuber♦
2 days ago
$begingroup$
It's a bit of a coincidence that the coefficients were not very different. Among other things, that requires the link function to be nearly the same as the identity function within the range of the explanatory variables.
$endgroup$
– whuber♦
2 days ago
add a comment |
2 Answers
2
active
oldest
votes
$begingroup$
If you take a look at the R help documentation you will note that there is no family
argument for the lm
function. By definition, lm
models (ordinary linear regression) in R are fit using ordinary least squares regression (OLS) which assumes the error terms of your model are normally distributed (i.e. family = gaussian
) with mean zero and a common variance. You cannot run a lm
model using other link functions (there are other functions to do that, though if you wanted--you just can't use lm
). In fact, when you try to run the lm
code you've presented above, R will generate a warning like this:
> > Warning message: In lm.fit(x, y, offset = offset, singular.ok =
> > singular.ok, ...) : extra argument ‘family’ is disregarded.
When you fit your model using glm
, on the other hand, you specified that the error terms in your model were binomial using a logit link function. This essentially constrains your model so that it assumes no constant error variance and it assumes the error terms can only be 0 or 1 for each observation. When you used lm
you made no such assumptions, but instead, your fit model assumed your errors could take on any value on the real number line. Put another way, lm
is a special case of glm
(one in which the error terms are assumed normal). It's entirely possible that you get a good approximation using lm
instead of glm
but it may not be without problems. For example, nothing in your lm
model will prevent your predicted values from lying outside $yin 0, 1$. So, how would you treat a predicted value of 1.05 for example (or maybe even trickier 0.5)? There are a number of other reasons to usually select the model that best describes your data, rather than using a simple linear model, but rather than my re-hashing them here, you can read about them in past posts like this one, this one, or perhaps this one.
Of course, you can always use a linear model if you wanted to--it depends on how precise you need to be in your predictions and what the consequences are of using predictions or estimates that might have the drawbacks noted.
$endgroup$
add a comment |
$begingroup$
Linear regression (lm
in R) does not have link function and assumes normal distribution. It is generalized linear model (glm
in R) that generalizes linear model beyond what linear regression assumes and allows for such modifications. In your case, the family
parameter was passed to the ...
method and passed further to other methods that ignore the not used parameter. So basically, you've run linear regression on your data.
$endgroup$
add a comment |
Your Answer
StackExchange.ready(function()
var channelOptions =
tags: "".split(" "),
id: "65"
;
initTagRenderer("".split(" "), "".split(" "), channelOptions);
StackExchange.using("externalEditor", function()
// Have to fire editor after snippets, if snippets enabled
if (StackExchange.settings.snippets.snippetsEnabled)
StackExchange.using("snippets", function()
createEditor();
);
else
createEditor();
);
function createEditor()
StackExchange.prepareEditor(
heartbeatType: 'answer',
autoActivateHeartbeat: false,
convertImagesToLinks: false,
noModals: true,
showLowRepImageUploadWarning: true,
reputationToPostImages: null,
bindNavPrevention: true,
postfix: "",
imageUploader:
brandingHtml: "Powered by u003ca class="icon-imgur-white" href="https://imgur.com/"u003eu003c/au003e",
contentPolicyHtml: "User contributions licensed under u003ca href="https://creativecommons.org/licenses/by-sa/3.0/"u003ecc by-sa 3.0 with attribution requiredu003c/au003e u003ca href="https://stackoverflow.com/legal/content-policy"u003e(content policy)u003c/au003e",
allowUrls: true
,
onDemand: true,
discardSelector: ".discard-answer"
,immediatelyShowMarkdownHelp:true
);
);
Cedroh is a new contributor. Be nice, and check out our Code of Conduct.
Sign up or log in
StackExchange.ready(function ()
StackExchange.helpers.onClickDraftSave('#login-link');
);
Sign up using Google
Sign up using Facebook
Sign up using Email and Password
Post as a guest
Required, but never shown
StackExchange.ready(
function ()
StackExchange.openid.initPostLogin('.new-post-login', 'https%3a%2f%2fstats.stackexchange.com%2fquestions%2f404401%2flm-and-glm-function-in-r%23new-answer', 'question_page');
);
Post as a guest
Required, but never shown
2 Answers
2
active
oldest
votes
2 Answers
2
active
oldest
votes
active
oldest
votes
active
oldest
votes
$begingroup$
If you take a look at the R help documentation you will note that there is no family
argument for the lm
function. By definition, lm
models (ordinary linear regression) in R are fit using ordinary least squares regression (OLS) which assumes the error terms of your model are normally distributed (i.e. family = gaussian
) with mean zero and a common variance. You cannot run a lm
model using other link functions (there are other functions to do that, though if you wanted--you just can't use lm
). In fact, when you try to run the lm
code you've presented above, R will generate a warning like this:
> > Warning message: In lm.fit(x, y, offset = offset, singular.ok =
> > singular.ok, ...) : extra argument ‘family’ is disregarded.
When you fit your model using glm
, on the other hand, you specified that the error terms in your model were binomial using a logit link function. This essentially constrains your model so that it assumes no constant error variance and it assumes the error terms can only be 0 or 1 for each observation. When you used lm
you made no such assumptions, but instead, your fit model assumed your errors could take on any value on the real number line. Put another way, lm
is a special case of glm
(one in which the error terms are assumed normal). It's entirely possible that you get a good approximation using lm
instead of glm
but it may not be without problems. For example, nothing in your lm
model will prevent your predicted values from lying outside $yin 0, 1$. So, how would you treat a predicted value of 1.05 for example (or maybe even trickier 0.5)? There are a number of other reasons to usually select the model that best describes your data, rather than using a simple linear model, but rather than my re-hashing them here, you can read about them in past posts like this one, this one, or perhaps this one.
Of course, you can always use a linear model if you wanted to--it depends on how precise you need to be in your predictions and what the consequences are of using predictions or estimates that might have the drawbacks noted.
$endgroup$
add a comment |
$begingroup$
If you take a look at the R help documentation you will note that there is no family
argument for the lm
function. By definition, lm
models (ordinary linear regression) in R are fit using ordinary least squares regression (OLS) which assumes the error terms of your model are normally distributed (i.e. family = gaussian
) with mean zero and a common variance. You cannot run a lm
model using other link functions (there are other functions to do that, though if you wanted--you just can't use lm
). In fact, when you try to run the lm
code you've presented above, R will generate a warning like this:
> > Warning message: In lm.fit(x, y, offset = offset, singular.ok =
> > singular.ok, ...) : extra argument ‘family’ is disregarded.
When you fit your model using glm
, on the other hand, you specified that the error terms in your model were binomial using a logit link function. This essentially constrains your model so that it assumes no constant error variance and it assumes the error terms can only be 0 or 1 for each observation. When you used lm
you made no such assumptions, but instead, your fit model assumed your errors could take on any value on the real number line. Put another way, lm
is a special case of glm
(one in which the error terms are assumed normal). It's entirely possible that you get a good approximation using lm
instead of glm
but it may not be without problems. For example, nothing in your lm
model will prevent your predicted values from lying outside $yin 0, 1$. So, how would you treat a predicted value of 1.05 for example (or maybe even trickier 0.5)? There are a number of other reasons to usually select the model that best describes your data, rather than using a simple linear model, but rather than my re-hashing them here, you can read about them in past posts like this one, this one, or perhaps this one.
Of course, you can always use a linear model if you wanted to--it depends on how precise you need to be in your predictions and what the consequences are of using predictions or estimates that might have the drawbacks noted.
$endgroup$
add a comment |
$begingroup$
If you take a look at the R help documentation you will note that there is no family
argument for the lm
function. By definition, lm
models (ordinary linear regression) in R are fit using ordinary least squares regression (OLS) which assumes the error terms of your model are normally distributed (i.e. family = gaussian
) with mean zero and a common variance. You cannot run a lm
model using other link functions (there are other functions to do that, though if you wanted--you just can't use lm
). In fact, when you try to run the lm
code you've presented above, R will generate a warning like this:
> > Warning message: In lm.fit(x, y, offset = offset, singular.ok =
> > singular.ok, ...) : extra argument ‘family’ is disregarded.
When you fit your model using glm
, on the other hand, you specified that the error terms in your model were binomial using a logit link function. This essentially constrains your model so that it assumes no constant error variance and it assumes the error terms can only be 0 or 1 for each observation. When you used lm
you made no such assumptions, but instead, your fit model assumed your errors could take on any value on the real number line. Put another way, lm
is a special case of glm
(one in which the error terms are assumed normal). It's entirely possible that you get a good approximation using lm
instead of glm
but it may not be without problems. For example, nothing in your lm
model will prevent your predicted values from lying outside $yin 0, 1$. So, how would you treat a predicted value of 1.05 for example (or maybe even trickier 0.5)? There are a number of other reasons to usually select the model that best describes your data, rather than using a simple linear model, but rather than my re-hashing them here, you can read about them in past posts like this one, this one, or perhaps this one.
Of course, you can always use a linear model if you wanted to--it depends on how precise you need to be in your predictions and what the consequences are of using predictions or estimates that might have the drawbacks noted.
$endgroup$
If you take a look at the R help documentation you will note that there is no family
argument for the lm
function. By definition, lm
models (ordinary linear regression) in R are fit using ordinary least squares regression (OLS) which assumes the error terms of your model are normally distributed (i.e. family = gaussian
) with mean zero and a common variance. You cannot run a lm
model using other link functions (there are other functions to do that, though if you wanted--you just can't use lm
). In fact, when you try to run the lm
code you've presented above, R will generate a warning like this:
> > Warning message: In lm.fit(x, y, offset = offset, singular.ok =
> > singular.ok, ...) : extra argument ‘family’ is disregarded.
When you fit your model using glm
, on the other hand, you specified that the error terms in your model were binomial using a logit link function. This essentially constrains your model so that it assumes no constant error variance and it assumes the error terms can only be 0 or 1 for each observation. When you used lm
you made no such assumptions, but instead, your fit model assumed your errors could take on any value on the real number line. Put another way, lm
is a special case of glm
(one in which the error terms are assumed normal). It's entirely possible that you get a good approximation using lm
instead of glm
but it may not be without problems. For example, nothing in your lm
model will prevent your predicted values from lying outside $yin 0, 1$. So, how would you treat a predicted value of 1.05 for example (or maybe even trickier 0.5)? There are a number of other reasons to usually select the model that best describes your data, rather than using a simple linear model, but rather than my re-hashing them here, you can read about them in past posts like this one, this one, or perhaps this one.
Of course, you can always use a linear model if you wanted to--it depends on how precise you need to be in your predictions and what the consequences are of using predictions or estimates that might have the drawbacks noted.
edited 2 days ago
duckmayr
1846
1846
answered 2 days ago
StatsStudentStatsStudent
6,24032144
6,24032144
add a comment |
add a comment |
$begingroup$
Linear regression (lm
in R) does not have link function and assumes normal distribution. It is generalized linear model (glm
in R) that generalizes linear model beyond what linear regression assumes and allows for such modifications. In your case, the family
parameter was passed to the ...
method and passed further to other methods that ignore the not used parameter. So basically, you've run linear regression on your data.
$endgroup$
add a comment |
$begingroup$
Linear regression (lm
in R) does not have link function and assumes normal distribution. It is generalized linear model (glm
in R) that generalizes linear model beyond what linear regression assumes and allows for such modifications. In your case, the family
parameter was passed to the ...
method and passed further to other methods that ignore the not used parameter. So basically, you've run linear regression on your data.
$endgroup$
add a comment |
$begingroup$
Linear regression (lm
in R) does not have link function and assumes normal distribution. It is generalized linear model (glm
in R) that generalizes linear model beyond what linear regression assumes and allows for such modifications. In your case, the family
parameter was passed to the ...
method and passed further to other methods that ignore the not used parameter. So basically, you've run linear regression on your data.
$endgroup$
Linear regression (lm
in R) does not have link function and assumes normal distribution. It is generalized linear model (glm
in R) that generalizes linear model beyond what linear regression assumes and allows for such modifications. In your case, the family
parameter was passed to the ...
method and passed further to other methods that ignore the not used parameter. So basically, you've run linear regression on your data.
answered 2 days ago


Tim♦Tim
60.7k9133230
60.7k9133230
add a comment |
add a comment |
Cedroh is a new contributor. Be nice, and check out our Code of Conduct.
Cedroh is a new contributor. Be nice, and check out our Code of Conduct.
Cedroh is a new contributor. Be nice, and check out our Code of Conduct.
Cedroh is a new contributor. Be nice, and check out our Code of Conduct.
Thanks for contributing an answer to Cross Validated!
- Please be sure to answer the question. Provide details and share your research!
But avoid …
- Asking for help, clarification, or responding to other answers.
- Making statements based on opinion; back them up with references or personal experience.
Use MathJax to format equations. MathJax reference.
To learn more, see our tips on writing great answers.
Sign up or log in
StackExchange.ready(function ()
StackExchange.helpers.onClickDraftSave('#login-link');
);
Sign up using Google
Sign up using Facebook
Sign up using Email and Password
Post as a guest
Required, but never shown
StackExchange.ready(
function ()
StackExchange.openid.initPostLogin('.new-post-login', 'https%3a%2f%2fstats.stackexchange.com%2fquestions%2f404401%2flm-and-glm-function-in-r%23new-answer', 'question_page');
);
Post as a guest
Required, but never shown
Sign up or log in
StackExchange.ready(function ()
StackExchange.helpers.onClickDraftSave('#login-link');
);
Sign up using Google
Sign up using Facebook
Sign up using Email and Password
Post as a guest
Required, but never shown
Sign up or log in
StackExchange.ready(function ()
StackExchange.helpers.onClickDraftSave('#login-link');
);
Sign up using Google
Sign up using Facebook
Sign up using Email and Password
Post as a guest
Required, but never shown
Sign up or log in
StackExchange.ready(function ()
StackExchange.helpers.onClickDraftSave('#login-link');
);
Sign up using Google
Sign up using Facebook
Sign up using Email and Password
Sign up using Google
Sign up using Facebook
Sign up using Email and Password
Post as a guest
Required, but never shown
Required, but never shown
Required, but never shown
Required, but never shown
Required, but never shown
Required, but never shown
Required, but never shown
Required, but never shown
Required, but never shown
FzGD7,A,I7 9O1yCUFxJ nJ16SRM
$begingroup$
Thank you both for your insight on this issue.
$endgroup$
– Cedroh
2 days ago
$begingroup$
no need to thank us in the comment -- simply up-vote answer you found helpful and select the check mark next to the one that best answered your question. Thanks! As a new user of this site, I just wanted to make sure you knew how to use these functions! You are welcome by the way.
$endgroup$
– StatsStudent
2 days ago
1
$begingroup$
It's a bit of a coincidence that the coefficients were not very different. Among other things, that requires the link function to be nearly the same as the identity function within the range of the explanatory variables.
$endgroup$
– whuber♦
2 days ago